Call for Participation
Causal AI for Robust Decision Making (CARD)
Monday, 23 June 2025, 08:30 - 12:30 CEST
Organizers
Adrienne Raglin
DEVCOM Army Research Laboratory, USA
adrienne.raglin2.civ@army.mil
Anjon Basak
Stormfish Scientific Corporation, USA
anjon.basak@stormfish.io
Aim of the Workshop
As AI becomes an integral part of our daily lives, we increasingly rely on its suggestions and automated systems to guide decision-making. From healthcare to autonomous driving and even financial planning, AI plays a vital role in helping humans navigate complex environments and make important choices. However, current AI systems are largely based on pattern recognition and correlations within data, which often limits their ability to reason about cause and effect. This reliance on correlation can lead to suboptimal recommendations, particularly in critical decision-making scenarios, where understanding the underlying causal dynamics is essential.
When AI is not grounded in causality, human-AI collaboration becomes riskier. For instance, AI may recommend actions based on patterns that seem effective but overlook key causal relationships, potentially leading to poor or even dangerous decisions. Whether in healthcare, emergency response, or transportation, such decisions could result in adverse outcomes, highlighting the urgent need for AI systems that can reason about causality rather than simply finding correlations.
Incorporating causal reasoning into AI systems will make them more robust, allowing AI to predict outcomes based on real-world cause-and-effect relationships. This will lead to AI systems that are not only more accurate but also more transparent and trustworthy, as they can convey the reasoning behind their recommendations to human users. Such systems will foster stronger collaboration by providing insights grounded in causality, leading to better decision-making in high-stakes environments.
For example, in autonomous driving, causal AI could help vehicles predict the behaviors of pedestrians and other drivers by understanding the causal links between environmental conditions, driver behavior, and accidents. This could drastically reduce accidents and improve road safety by ensuring that decisions are based on more than just observed patterns. This insight can also be utilized by humans to make better decisions and build a trustworthy relationship with the causal AI.
The aim of this workshop is to explore and advance the development of causal reasoning in AI systems to improve interpretability, transparency, decision-making, enhance human-AI collaboration, and increase the robustness, safety and reliability of AI in high-stakes environments. The workshop seeks to address the limitations of correlation-based AI by promoting frameworks and methodologies that integrate causality, enabling AI systems to reason about cause-and-effect relationships, provide actionable insights, and build trust with human users across various domains.
Expected Workshop outcome
- Frameworks for Causal Reasoning in AI and ML:
Development of innovative tools and methodologies for causal analysis in diverse scenarios like autonomous driving, emergency response, and healthcare. - Contributions to Foundational Concepts:
Exploration of core concepts, including causal models, causal graphs, interventions, counterfactuals, and causal inference, and their integration into AI systems. - Improved Human-AI Collaboration Through Causal Transparency:
Methods for effective communication of causal insights from AI to users through interpretability and explainability, facilitating better decision-making and situational awareness. - Counterfactual Evaluation Mechanisms in AI:
Application of counterfactual analysis to assess the effectiveness and reliability of causality-aware AI systems and visualize them using interpretability methods. - Comparative Use Case Analysis:
Insights from use cases demonstrating the benefits of causal reasoning over traditional machine learning approaches. - Addressing Challenges and Limitations:
Identification of key challenges, limitations, and future directions for implementing causality-aware AI.
Workshop topics
Foundations of Causal AI:
- Causal models and graphs.
- Interventions and counterfactuals.
- Causal inference in AI systems.
Applications of Causal Reasoning in AI:
- Autonomous driving and self-driving vehicles.
- Emergency response and disaster management.
- Healthcare diagnostics and personalized treatment.
- Urban planning, traffic management, and infrastructure design.
- Education and adaptive learning systems.
- Finance and risk assessment.
Counterfactual Analysis and Evaluation:
- Techniques for simulating alternative scenarios.
- Metrics for evaluating the impact of causally-aware AI.
Challenges and Future Directions:
- Limitations of current causal models in real-world applications.
- Scalability and computational challenges.
- Improving interpretability and transparency in causal AI systems.
Workshop agenda
The following is a framework for the program of the Workshop:
Time |
Program event |
---|---|
08:30 - 08:45 |
Introduction and context
|
08:45 - 09:15 |
Presentations on foundational concepts: causal models, graphs, interventions, and counterfactuals. Causal inference techniques for AI. Interactive Q&A on challenges and opportunities. |
9:15 - 10:30 |
Presentations on case studies in disaster response, autonomous driving, healthcare, and urban planning. Applications in finance, education, and disaster response. Panel discussion on cross-domain insights and lessons learned Presentations on counterfactual techniques and their applications. |
10:30 - 11:00 |
Break |
11:00 - 12:30 |
Hands-on activity: Designing counterfactual scenarios for selected AI tasks. Presentations on challenges in scalability, computational limitations, and deployment. Collaborative brainstorming session on future research and solutions. Next steps, call for collaboration, and acknowledgments |
Guidelines to prospective authors
Submission for the Workshop
Prospective authors should submit their proposals in PDF format through the HCII Conference Management System (CMS). We accept the following forms of submission
Long-Form Papers:
- Full research papers up to 10-20 pages (excluding references).
Short-Form Papers:
- Concise papers up to 4–11 pages (excluding references).
Submission for the Conference Proceedings
The contributions to be presented in the context of Workshops will not be automatically included in the Conference proceedings.
However, after consultation with the Workshop organizer(s), authors of accepted Workshop proposals who are registered for the Conference are welcome to submit, through the Conference Management System (CMS), an extended version of their Workshop contribution to be considered, following further peer review, for presentation at the Conference and inclusion in the “Late Breaking” volumes of the Conference proceedings, either in the LNCS as a long paper (typically 12 pages, but no less than 10 and no more than 20 pages), or in the CCIS as a short paper/extended poster abstract (typically 6 pages, but no less than 4 and no more than 11).
Workshop deadlines
Submission Deadline: contributions |
March 15, 2025 |
Notification of Acceptance: |
April 10, 2025 |
Camera-Ready Submission: |
April 25, 2025 |
Finalization of Workshop organization and registration of participants |
May 2, 2025 |
Workshop organizers
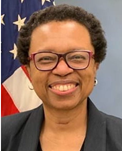
Dr. Adrienne Raglin
adrienne.raglin2.civ@army.mil
Dr. Adrienne Raglin is an Electronics Engineer at the DEVCOM Army Research Laboratory (ARL), serving in the Army Research Directorate's Military Information Sciences Division, Content Understanding Branch. She earned her Ph.D. in Electrical Engineering from Howard University in 2003, following her M.S. and B.S. in Electrical Engineering from the Georgia Institute of Technology in 1991 and 1989, respectively. She also holds a B.S. in Computer Science, awarded in 1989 by Spelman College.
Dr. Raglin's scientific interests encompass image processing, information uncertainty, human-information interaction, and artificial reasoning. She actively collaborates with academia, industry, and other organizations, alongside ARL researchers, to tackle the complexities and challenges associated with enhancing command and control and improving decision-making processes.
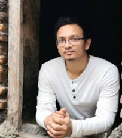
Anjon Basak
anjon.basak@stormfish.io
Anjon Basak earned his PhD in Computer Science in 2020 from the University of Texas at El Paso. He is an experienced AI/ML Research Scientist specializing in large vision models, vision-language models, computer vision, and generative AI. Anjon's expertise includes interpretability, causal reasoning, counterfactuals, and uncertainty quantification.
He has a proven track record of leading innovative projects, mentoring teams, and developing scalable frameworks for explainability, bias mitigation, and robust decision-making in complex AI systems. Currently, Anjon supports the Artificial Reasoning Team at DEVCOM ARL as a contractor, where he contributes to advancing AI technologies in critical domains.
Useful links and References
Causal AI conference https://conference.causalens.com/
Registration regulation
Workshops will run as 'hybrid' events. Organizers are themselves expected to attend ‘on-site’, while participants will have the option to attend either 'on-site' or 'on-line'. The total number of participants per Workshop cannot be less than 8 or exceed 25.
Workshops are ‘closed’ events, i.e. only authors of accepted submissions for a Workshop will be able to register to attend the specific Workshop, complimentary with their Conference registration.